Real-time Optimal Price Selection from Multiple Forex Sources
In foreign exchange (forex) market, prices for the same instrument at the same time may vary. Real-time selection of the optimal price among these sources is crucial as it helps investors achieve more favorable execution prices, reduce transaction costs, optimize liquidity, and diversify transaction risks. For high-frequency or algorithmic traders, acquiring optimal prices from various sources can lead to more effective execution of trading strategies. Furthermore, by comparing quotes from different price sources, investors can better grasp market trends and volatility, and make more informed bid price investment decisions.
This tutorial demonstrates how to implement real-time optimal price selection based on DolphinDB stream processing framework.
1. Overview
This case uses CFETS (China Foreign Exchange Trade System) and HSBC as examples to illustrate how to achieve optimal prices selection among different price sources, while also handling anomalous data. The specific rules are as follows:
- Set a threshold for the bid prices of each currency pair, where prices exceeding this threshold are considered anomalous.
- If the bid prices from both markets do not exceed the threshold, select the larger bid price as the optimal price.
- If one market's bid price exceeds the threshold, select the bid price from the other market as the optimal price.
- If both markets' bid prices exceed the threshold, return the optimal price from the previous moment for that currency pair.
This case is implemented using an equi join engine and a reactive state engine. The workflow is as follows:

The data processing is as follows:
- Data from the two markets enters the equi join engine through two stream tables.
- The equi join engine selects the optimal price and market for the currency pair at a given time; if both market prices are anomalies, it sets the optimal price and market for that moment to null.
- The results from the equi join engine are injected into the reactive state engine, where null values for optimal prices and markets are forward filled.
- The final results are output in real-time to a stream table.
- Application systems can subscribe to this result stream table to obtain real-time optimal prices for trading guidance, risk control, and visualization.
2. Forex Data Simulation
This case simulates real-time forex data by replaying generated bid data for a day from CFETS and HSBC. The data structure has been simplified for this example, focusing solely on bid quotes. The detailed schema of data is illustrated in the figure below:
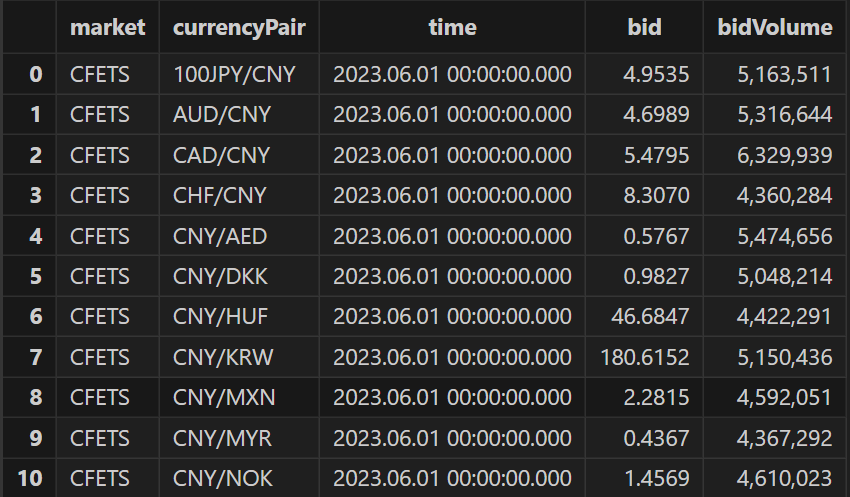
To make the simulated data more reflective of actual market conditions, we utilize the bid quotes from the forex trading center for 29 common currency pairs at a specific time point. Each bid price serves as the mean of a normal distribution, with a standard deviation of 0.1, to generate simulated data that conforms to this distribution. To filter out anomalous quotes, an abnormal threshold is set by adding 0.08 to each currency pair's real value. Data is generated for each currency pair every 500 milliseconds.
The code for generating simulated data is as follows:
def genOneDayData(rawCurrencyPair,rawBid,date,marketName)
{
dataTable = table(1:0,`market`currencyPair`time`bid`bidVolume,[`SYMBOL,`SYMBOL,`TIMESTAMP,"DOUBLE","INT"])
num = 0
for(i in 0..(rawCurrencyPair.size() - 1))
{
market = take(marketName,172800)
currencyPair = take(rawCurrencyPair[i],172800)
time = concatDateTime(date,00:00:00.000 + 500*(0..(172800-1)))
bid = norm(rawBid[i],0.1,172800)
bidVolume = int(norm(5000000,500000,172800))
t = table(market,currencyPair,time,bid,bidVolume)
dataTable.append!(t)
}
return select * from dataTable order by time,currencyPair
}
// The real data copied from the official website of CFETS
currencyPair = ["NZD/USD","USD/JPY","USD/CHF","GBP/USD","EUR/JPY","CNY/THB","CNY/TRY","CNY/SEK","CNY/PLN","CNY/SAR","CNY/KRW","CNY/RUB","CAD/CNY","SGD/CNY","AUD/CNY","HKD/CNY","EUR/CNY","CNY/MXN","CNY/NOK","CNY/DKK","CNY/HUF","CNY/AED","CNY/ZAR","CNY/MYR","CHF/CNY","NZD/CNY","GBP/CNY","100JPY/CNY","USD/CNY"]
bids = [0.61114,143.984,0.89425,1.27282,157.7,4.904,3.60415,1.4865,0.56205,0.51888,180.72,11.5679,5.4696,5.3465,4.7983,0.92282,7.9141,2.365,1.4956,0.9407,46.746,0.50849,2.5687,0.644,8.0801,4.4165,9.198,5.0189,7.2262]
date = 2023.06.01
// Create HSBC tick data
HSBC_Tick = genOneDayData(currencyPair,bids,date,`HSBC)
// Create CFETS tick data
CFETS_Tick = genOneDayData(currencyPair,bids,date,`CFETS)
//Set thresholds
threshold = dict(currencyPair,bids + 0.08)
The simulated data is stored in two in-memory tables: CFETS_Tick and HSBC_Tick. In the subsequent steps, this data will be replayed into the corresponding stream tables to simulate real-time market conditions. The thresholds for determining whether the bid prices of the 29 currency pairs are anomalous are stored in a dictionary variable called “threshold”, where the key is the currency pair and the value is the threshold for that currency pair.
3. Implementation
In this section, we will focus on how to implement the proposed solution, detailing the necessary steps and code required to achieve real-time optimal price selection from multiple currency sources.
3.1 Environment Preparation and Stream Table Creation
The environment must first be cleaned, and the necessary stream tables must be created to receive and process the forex market data.
def cleanEnvironment(){
try{ unsubscribeTable(tableName = `HSBCStreamTable, actionName = "joinLeft") }catch(ex){ print(ex) }
try{ dropStreamTable(`HSBCStreamTable)}catch(ex){ print(ex) }
try{ unsubscribeTable(tableName = "CFETSTStreamTable", actionName = "joinRight")}catch(ex){ print(ex) }
try{ dropStreamTable(`CFETSTStreamTable)}catch(ex){ print(ex) }
try{ dropStreamTable(`bestPriceStreamTable)}catch(ex){ print(ex) }
try{ dropStreamEngine(`bestPricEngine)}catch(ex){ print(ex) }
try{ dropStreamEngine(`fillPrevEngine)}catch(ex){ print(ex) }
}
cleanEnvironment()
def createStreamTableFunc()
{
// Define streamTables that receive original market data: HSBCStreamTable and CFETSTStreamTable
colName = `market`currencyPair`time`bid`bidVolume
colType = [`SYMBOL,`SYMBOL,`TIMESTAMP,"DOUBLE","INT"]
HSBCTemp = streamTable(20000000:0,colName,colType)
try{ enableTableShareAndPersistence(table = HSBCTemp, tableName = "HSBCStreamTable", asynWrite = true, compress = true, cacheSize = 20000000, retentionMinutes = 1440, flushMode = 0, preCache = 10000) }
catch(ex){ print(ex) }
CFETSTemp = streamTable(20000000:0,colName,colType)
try{ enableTableShareAndPersistence(table = CFETSTemp, tableName = "CFETSTStreamTable", asynWrite = true, compress = true, cacheSize = 20000000, retentionMinutes = 1440, flushMode = 0, preCache = 10000) }
catch(ex){ print(ex) }
// Define streamTables that save results: bestPriceTempStreamTable
colName = `currencyPair`time`market`bestPrice`leftBid`rightBid`delay
colType = [`SYMBOL,`TIMESTAMP,`INT,`DOUBLE,`DOUBLE,`DOUBLE,`DOUBLE]
bestPriceTemp = streamTable(20000000:0,colName,colType)
try{ enableTableShareAndPersistence(table = bestPriceTemp, tableName = "bestPriceStreamTable", asynWrite = true, compress = true, cacheSize = 20000000, retentionMinutes = 1440, flushMode = 0, preCache = 10000) }
catch(ex){ print(ex) }
}
createStreamTableFunc()
go
The above script calls enableTableShareAndPersistence to create three shared persisted tream tables. The “HSBCStreamTable” and “CFETSTStreamTable” are used to receive and publish real-time market data from HSBC and CFETST, respectively. The “bestPriceStreamTable” is used to receive and publish the output results table after processing by the engine.
Note: To ensure the successful execution of the
enableTableShareAndPersistence
command, it is necessary to
first configure the configuration parameter persistenceDir in the
configuration file (dolohindb.cfg in standalone mode and
cluster.cfg in cluster mode). For details of this configuration
parameter, see Reference.
3.2 Optimal Price and Market Function Definition
Define a function to implement the selection of the optimal priceand market. All input parameters of this function, except for the threshold, are vectors corresponding to the columns in the “tick” table. The threshold represents the anomaly thresholds for each currency pair generated from the simulated data. This function will later be specified as a parameter in the equi join engine.
def bestPriceAndMarket(leftBid, rightBid, leftMarket, rightMarket, currencyPair, threshold)
{
price = iif(leftBid > threshold[currencyPair] and rightBid > threshold[currencyPair],NULL,iif((leftBid > threshold[currencyPair]),rightBid,iif(rightBid > threshold[currencyPair],leftBid,max(leftBid,rightBid))))
market = iif(leftBid > threshold[currencyPair] and rightBid > threshold[currencyPair],NULL,iif((leftBid > threshold[currencyPair]),2,iif(rightBid > threshold[currencyPair],1,iif(leftBid > rightBid,1,2))))
return market, price
}
In the code above:
- The statement
iif(cond, trueResult, falseResult)
indicates that if the condition cond is met, it returns trueResult; otherwise, it returns falseResult. This is equivalent to runningif(cond[i]) truResult(cond[i]) else falseResult(cond[i])
statement. - To facilitate subsequent data filling, the market type is converted to an integer, with the left table returning 1 and the right table returning 2.
3.3 Streaming Engine Creation and Stream Table subscription
We implement this solution by combining the equi join engine and the reactive state engine into a pipeline, where the output of the equi join engine serves as the input for the reactive state engine. The code is as follows:
fillPrevSch = table(1:0,`time`currencyPair`market`bestPrice`leftBid`rightBid`inTime,[`TIMESTAMP,`SYMBOL,`INT,`DOUBLE,`DOUBLE,`DOUBLE,`NANOTIMESTAMP])
// create reactiveStateEngine
fillPrevEngine = createReactiveStateEngine(name = "fillPrevEngine", metrics = [<Time>,<ffill(Market)>,<ffill(BestPrice)>,<leftBid>,<rightBid>,<(now(true) - inTime) \ 1000000>], dummyTable = fillPrevSch, outputTable = bestPriceStreamTable, keyColumn="CurrencyPair")
HSBC_Table_left = table(1:0,`market`currencyPair`time`bid`bidVolume`inTime,[`SYMBOL,`SYMBOL,`TIMESTAMP,`DOUBLE,`INT,`NANOTIMESTAMP])
CFETS_Table_right = table(1:0,`market`currencyPair`time`bid`bidVolume`inTime,[`SYMBOL,`SYMBOL,`TIMESTAMP,`DOUBLE,`INT,`NANOTIMESTAMP])
// create equiJoinEngine
bestPricEejEngine = createEquiJoinEngine(name = "bestPricEngine", leftTable = HSBC_Table_left, rightTable = CFETS_Table_right, outputTable = fillPrevEngine, metrics = [<bestPriceAndMarket(HSBC_Table_left.bid,CFETS_Table_right.bid,HSBC_Table_left.market,CFETS_Table_right.market,currencyPair,threshold) as `market`bestPrice>,<HSBC_Table_left.bid>,<CFETS_Table_right.bid>,<max(HSBC_Table_left.inTime,CFETS_Table_right.inTime)>], matchingColumn = `currencyPair, timeColumn = `time)
def appendTime(engine,isLeftTable,mutable data)
{
data[`inTime] = now(true)
appendForJoin(engine,isLeftTable,data)
}
subscribeTable(tableName="HSBCStreamTable", actionName="joinLeft", offset=0, handler=appendTime{bestPricEejEngine, true,}, msgAsTable=true)
subscribeTable(tableName="CFETSTStreamTable", actionName="joinRight", offset=0, handler=appendTime{bestPricEejEngine, false,}, msgAsTable=true)
In the code above:
- The table variable "fillPrevSch" specifies the input table schema of the reactive state engine, and its column types and order must match exactly with those of the output table (outputTable) of the equi join engine.
- In the metrics of the reactive state engine, the
ffill
function is specified to forward fill null values. Non-empty previous values for the same currency pair are used for filling. - The parameter outputTable of the equi join engine is specified as the reactive state engine ("fillPrevEngine").
- The function
bestPriceAndMarket
, which selects the optimal price and market, is specified in the parameter metrics of the equi join engine. - The user-defined function
appendTime
reserves a time column (in nanoseconds) to record the timestamp of data ingested into the equi join engine, facilitating subsequent delay statistics.
3.4 Subscription of Optimal Price Results
We can subscribe to the output table of the reactive state engine (optimal price results) through APIs, plugins, or other methods for operations such as visualization and monitoring.
3.4.1 Python API Subscription
By subscribing to the result table through the Python API, we can obtain the real-time optimal prices and their markets. The code is as follows:
import dolphindb as ddb
import numpy as np
from threading import Event
def resultProcess(lst):
print(lst)
s = ddb.session()
s.enableStreaming()
s.subscribe(host = "192.168.100.3", port = 8848, handler = resultProcess, tableName = "bestPriceStreamTable", actionName="pySub", offset=-1, resub=False)
Event().wait()
- Before executing the Python code, you must first define the stream table “bestPriceStreamTable” on the DolphinDB server.
- The
enableStreaming
method is used to enable streaming data subscription. - The
subscribe
method is used to subscribe to DolphinDB’s stream tables. Its parameter handler is specified as the callback functionresultProcess
to print the real-time data received. Please refer to Subscription for more details. - Replace "host" in above code with your available IP address.
3.4.2 Excel Subscription for Real-Time Optimal Price
The DolphinDB Excel plugin allows real-time calculation results to be pushed to Excel, enabling Excel to access data from shared stream tables.

Right-click on the "bestPriceStreamTable" and select Export by Subscribe. A dialog box will pop up asking for the primary key, as shown in the image below:
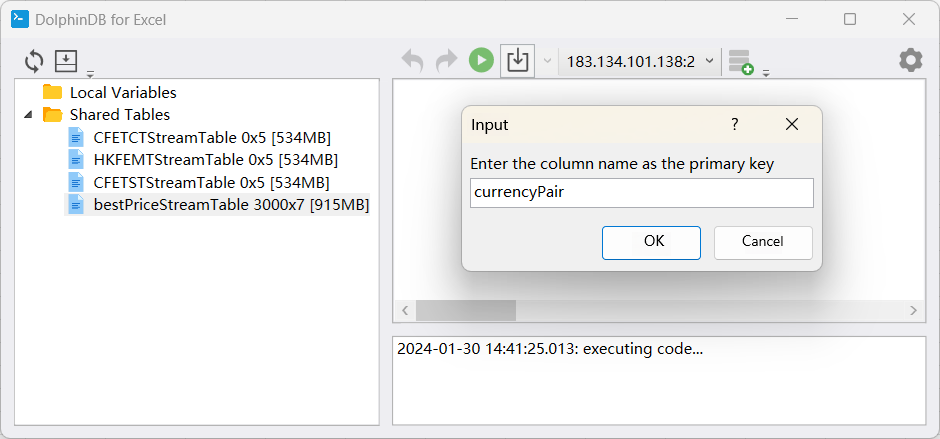
Enter the currency pair column "currencyPair" in the dialog box and click OK to complete the subscription.
3.4.3 Grafana Subscription for Real-Time Optimal Price
DolphinDB provides a Grafana data source plugin, allowing users to interact with DolphinDB through the Grafana dashboard by writing query scripts and subscribing to stream tables (through WebSocket) for data visualization. This section introduces the methods for monitoring in Grafana. Please refer to Grafana Datasource for a detailed tutorial.
The Query code in Grafana demonstrates the optimal price of the currency pair USD/CNY.
select gmtime(time) as ts, bestPrice from bestPriceStreamTable where currencyPair='USD/CNY' context by second(time) limit -1
Since Grafana displays UTC time by default, there may be a time
difference with the data time in the DolphinDB server. Therefore, you
need to use the gmtime
function for time zone
conversion in Grafana.
3.5 Data Replay
In this section, we implement the replay of market data and simulate real-time data writing. The code is as follows:
//replay data
submitJob("replayL","replayToLeft",replay,CFETS_Tick,`CFETSTStreamTable,`Time,,100)
submitJob("replayR","replayToRight",replay,HSBC_Tick,`HSBCStreamTable,`Time,,100)
t = getRecentJobs(2)
After execution, check the information in the variable "t":

If the "endTime" and "errorMsg" fields are empty, it indicates that the task is running as expected.
4. Computing Performance
The performance statistics account for the time taken from when the equi-join engine receives data to when the reactive state engine outputs results. The testing environment for this case is as follows (for reference only):
- CPU: Intel(R) Xeon(R) Silver 4210R CPU @ 2.40GHz
- Memory: 256GB
- Disk: SSD 3.5TB * 4
- OS: CentOS Linux release 7.6.1810
In this case, the maximum delay scenario occurs when 29 currency pairs arrive at the engine simultaneously, while the minimum delay scenario occurs with only 1 currency pair arriving at the engine. The delay statistics for single-threaded execution are shown in the table below:
Number of Currency Pairs | Delay (Milliseconds) |
---|---|
1 | 0.0841 |
29 | 0.1185 |
5. Summary
This tutorial is based on the DolphinDB stream processing framework and provides an efficient solution for real-time selection of the optimal price from multiple foreign exchange market sources. It aims to assist developers in using the DolphinDB stream processing framework to develop business applications, thereby reducing development complexity.
6. Appendix
Please execute each file in order according to the file numbers.